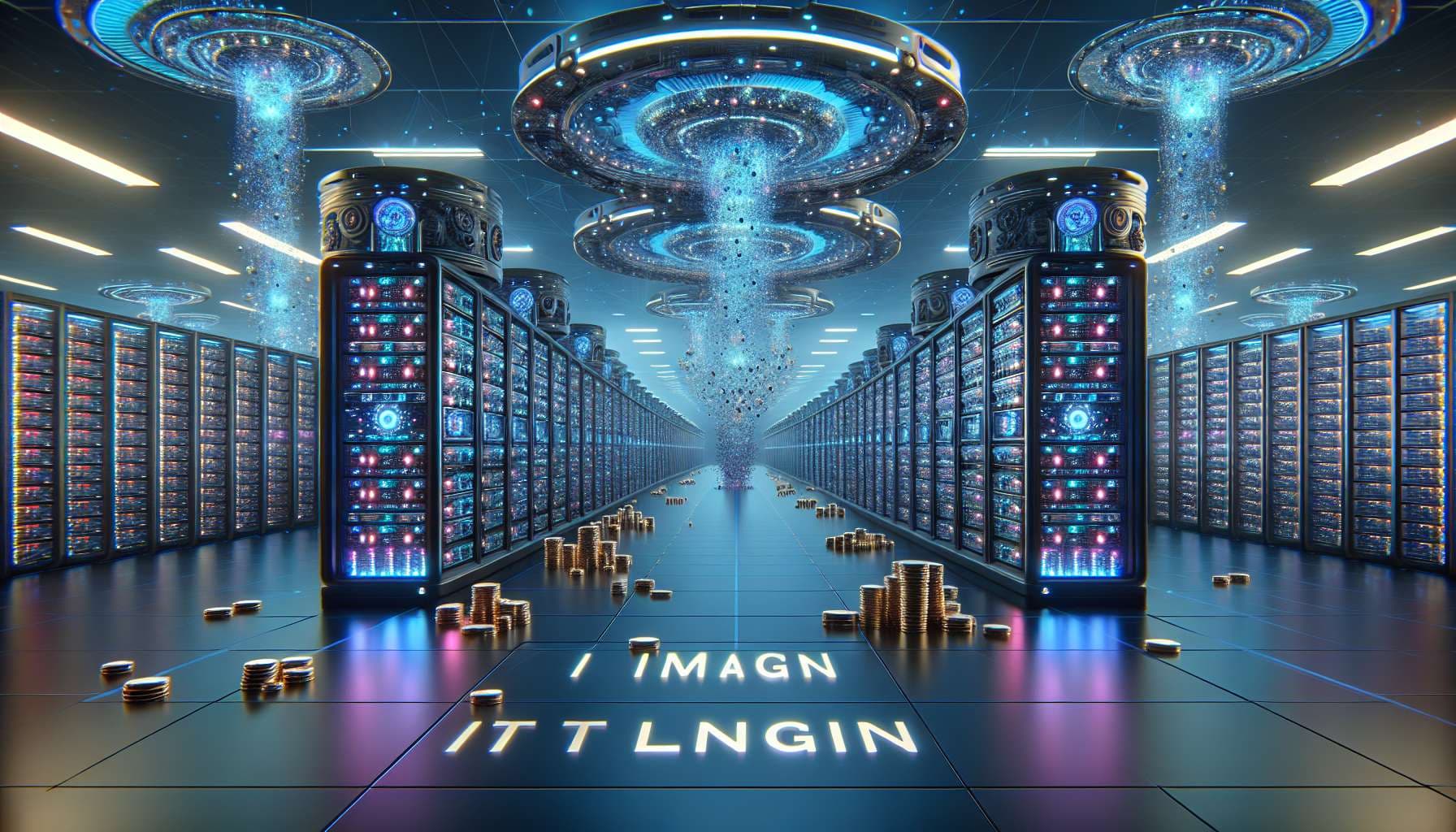
In "Situational Awareness: The Decade Ahead", Leopold Aschenbrenner estimates that the largest training clusters will cost over one trillion dollars around 2030.
Clarifications:
$1T worth of computers and associated data center infrastructure (e.g. building, cooling, networking; does not include the cost of the power plant)
Computers must be networked together and training one model. They do not need to synchronize weights at each gradient step.
Value of data centers will be estimated with reasonable depreciation. So, $1T of purchase price in TPUv1s would not count.
Nominal dollars.
So, if the nominal value of the datacenter being used to train a model less depreciation ever crosses $1T, this market resolves YES.
This is one of a series of markets on claims made in Leopold Aschenbrenner's Situational Awareness report(s).
Other markets about Leopold's predictions:
@wrhall I’ll count “$1T worth of computers, networked together, training one model”. A few details:
Value of data centers will be estimated with reasonable depreciation. So, $1T of purchase price in TPUv1s would not count.
$1T nominal dollars
@Tossup Seems fair! I don't know where they'll get the money (how big is their war chest?), but if you count 2 generations of tpus it feels plausible. Not sure if we will be able to get accurate accounting from them in 2030, but let's see